Complete List of Google Ranking Factors in 2025: How to Improve Your SEO
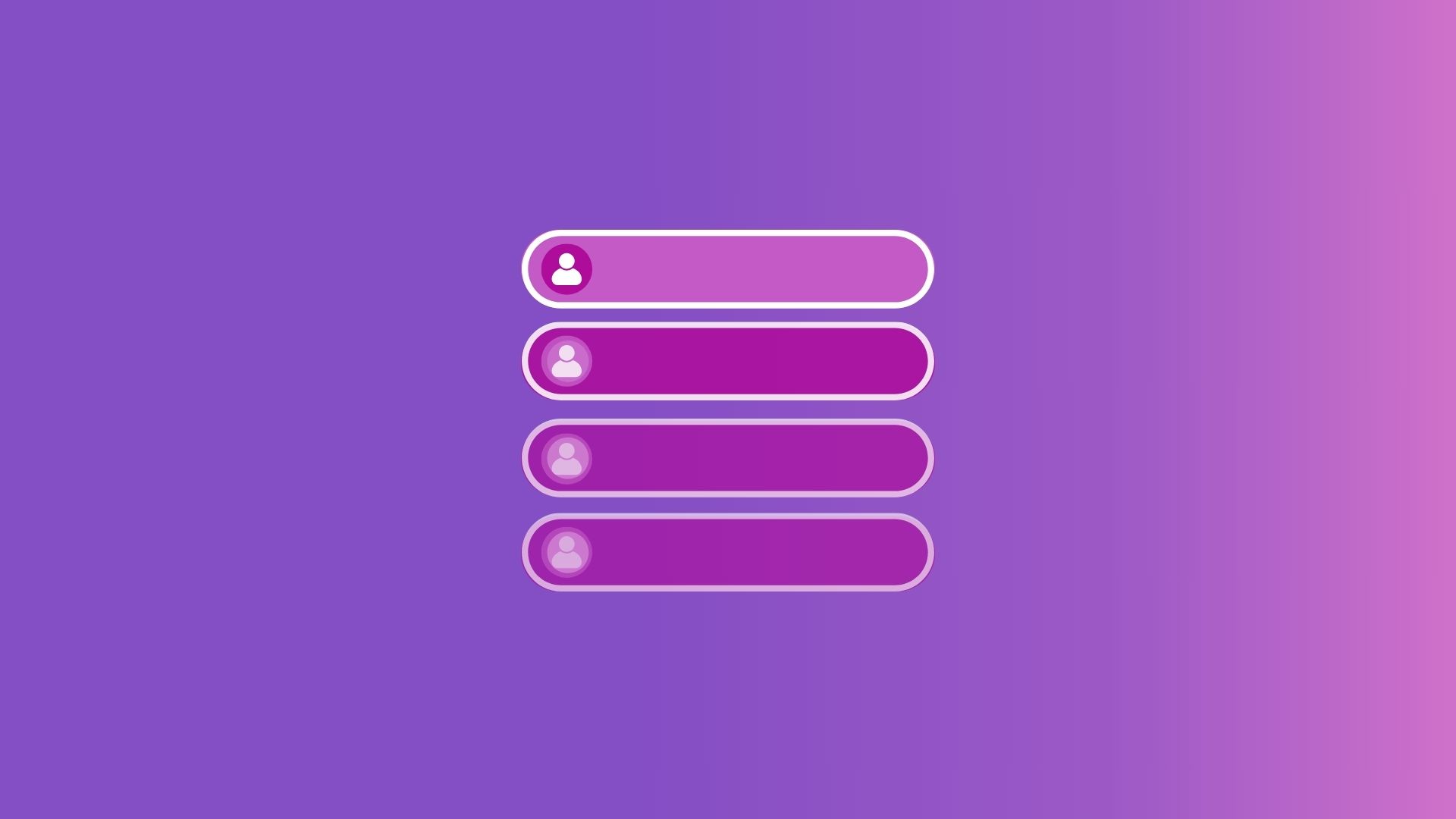
What Are Google Ranking Factors?
Google ranking factors are evolving elements that influence Google’s search engines. These are composed of algorithms and signals that respond to search queries. The purpose of ranking factors is to show relevant and informative search results.
It’s for this reason that websites optimize their SEO strategy. Over 60% of SEO professionals say that they’re satisfied with search results. Optimizing for ranking factors increases your web page performance.
How exactly influential are these ranking factors? Here’s how they affect the rankings of web pages:
- Relevance: Does the website display relevant content to the user query?
- Trustworthiness: Does the website offer factual and trustworthy content?
- Technical health: How quick and responsive is the website on different devices?
- Backlinks: Are there highly authoritative websites linking to the page?
The challenge is to know the current ranking factors. Google isn’t very keen on just providing this information.
To be safe, web pages try to cover all bases. They conduct comprehensive SEO audits throughout the year. Moreover, they benchmark their performance against the industry standards.
Having said that, recent reports point to essential factors in Google’s ranking systems. We’ll discuss these factors in detail in the following sections.
Top Google Search Ranking Factors in 2025
In 2025, these Google Search ranking factors hold the most weight in different niches.
Content quality
Google’s automated ranking systems prioritize original content. Search engines rank content that’s not just created for search rankings. The most important aspect is to have reliable, people-first content.
Websites boost their SEO campaigns through content optimization. But it doesn’t just mean producing more content than your competitors. You produce original content that answers users’ queries.
Along with originality, here are more tips for your content strategy:
- E-E-A-T: This covers Experience, Expertise, Authoritativeness, and Trustworthiness. The E-E-A-T approach is still relevant in SEO content strategy. Build up on author expertise and your website’s domain authority.
- Semantic search: Keyword stuffing doesn’t work anymore. Google’s introduction of a semantic search algorithm made sure of that. Now, content quality delves into the context of a search query. The more answers you provide, the more quality content you offer.
- Comprehensiveness: How does a page’s content cover the user’s search intent? This is an extension of semantic search. Did you discuss the topic comprehensively? Or did you just stuff it with keywords for search results?
It’s not about content length or quantity. Producing quality content requires a deeper understanding of user pain points. Know your audience and create helpful supplementary content.
Backlinks
You get “votes of trust” when you acquire backlinks from other sites. This increases your authority and trustworthiness in the industry. Therefore, backlinks rank as one of the important Google ranking factors.
However, be careful in acquiring links from other sites. Google’s spam policies double down on manipulative and spammy links.
Search engines encourage the use of white-hat link-building. The white-hat strategy integrates natural forms of link acquisition. These “natural” backlinks act as an excellent ranking signal on search results.
So, how do you acquire high-quality backlinks? We recommend these steps:
- First, create industry-specific content. Experiment with different forms, including whitepapers, case studies, and news. These types of content increase your website’s authority.
- Then, consider guest posting on websites in your niche. Research their audience and the topics they address. Contribute guest posts that provide helpful information to their audience.
- Next, acquire backlinks through directories and business listings. Google My Business is one example of a local directory. However, don’t give in to low-quality directory links. Ensure that links are coming from reputable directories.
Building a backlink profile focuses more on quality than quantity. Don’t interact with spammy sites. Aim for backlinks that you can get from relevant, high-authority websites.
Mobile-friendliness
In 2023, there were 6.5 billion active mobile internet users.
Moreover, 59.45% of global online traffic is attributed to mobile devices. More people are using smartphones to access search engine results.
So, it’s only right that you optimize for mobile usability. Here are the best practices for mobile optimization:
- Responsiveness: Your page should be responsive to any user interaction. It should seamlessly fit into various screen sizes.
- Speed: The average mobile internet loading speed is 8.6 seconds. But you should aim for faster site speed than that. We recommend minimal placements of ads and multimedia elements. These significantly contribute to the weight of your own website. In turn, it takes more time to fully load on mobile gadgets.
- Navigation: Can mobile users easily click your buttons and menus? Expect that your interface will change on mobile systems. You can simulate screen sizes on Chrome DevTools. Here, you can debug any navigation issues.
- Readability: Use very clear fonts and sizes. Users should be able to read your content even when they’re outside.
We recommend using tools like Google Search Console. This free tool inspects any mobile issues or delays that you might have.
Page speed
Now, let’s dig deeper into page loading speed.
We previously discussed mobile loading speed. On the desktop, the times get quicker. The average desktop internet loading time is 2.5 seconds.
Anything significantly longer than the average loading speed is detrimental. You’re risking potential engagements and conversions. Furthermore, you’re risking your rankings on search results.
Don’t worry, though. There are tools you can use to measure loading speed. Here are examples:
- Google PageSpeed Insights: Delves into Core Web Vitals to analyze UX elements. This tool also provides running analysis and continuous updates.
- GTmetrix: GTmetrix tests website speed on multiple testing locations. It allows you to test your loading speed in different countries. This is perfect for websites with global audiences.
- Pingdom: Pingdom isn’t just a speed-measuring tool. It also monitors historical performance and UX elements.
- WebPageTest: WebPageTest provides visual comparisons in videos. You can check your site’s performance over time. Also, this tool conducts data security and privacy checks.
Loading speed is an important factor in search engine results pages. Use speed measuring tools to discover which areas you can optimize.
User experience (UX)
UX is one most important ranking factors. The name says so itself. You’re optimizing your website for a smooth user experience.
However, many sites still fumble this SEO aspect. It’s not just about the content or the products you show. It’s also about how users can efficiently interact with your website.
User satisfaction is an essential Google ranking factor. We recommend these tips to ensure excellent UX:
- First, have real people test your website. This way, you can resolve issues before the full launch.
- Next, check your website’s mobile adaptability. Use Chrome DevTools to simulate different screen sizes.
- Prioritize speeding up the loading process. Refrain from placing too many ads or multimedia.
- Make clear navigation labels. Use color combinations that are easily readable.
- Be consistent with your company branding. Every page should adhere to your branding elements.
Moreover, ensure that you integrate accessibility guidelines. Follow Google’s Web Content Accessibility Guidelines (WCAG). This includes certain guidelines on alternative text and visual alignments.
Secure and accessible website
Data security is an SEO ranking factor that websites tend to overlook. Google doesn’t just focus on user satisfaction through quality and relevant results. They also want to protect their users.
That’s why security protocols are important in Google rankings. Here are the safety measures that Google expects from publishers:
- HTTPS: This stands for Hypertext Transfer Protocol Secure. It’s an encryption method to protect confidential user data. This helps prevent data breaches on passwords and financial information.
- Privacy compliance: Comply with regulations like GDPR and CCPA. These are regulations that focus on data privacy.
- Accessibility: Create inclusive designs and UX. Google’s algorithm prioritizes websites with accessibility features.
You can also add other security measures to safeguard user data. Many websites implement password policies and two-factor authentication (2FA).
Domain age, URL, and authority
Domain age and authority are also prioritized by search engine spiders. Of course, it doesn’t mean that older domains automatically rank on the first page. A successful domain should have good content and backlink profiles.
How does a website increase its authority in Google and other search engines? Here are several proven tips:
- Build a backlink profile of relevant and high-authority links. Don’t engage in spammy or black-hat link-building.
- Create a library of original content. Nothing builds authority better than ranking in organic search results.
- Take care of your website’s technical health. Monitor loading speed and mobile-friendliness. Fix or avoid any potential broken or duplicate links.
Moreover, it’s important that you optimize your URL. The structure should be concise but descriptive. Add the web page’s keyword and branding to your URL.
Technical SEO
The technical health of your website is a telling factor in Google. Ultimately, Google prioritizes websites with little to zero issues. This is heightened even more when it comes to user experience.
For example, you have a real good, quality content. However, your page takes a long time to load because of heavy multimedia elements. This will drive away your website visitors.
In turn, you accumulate higher bounce rates and shorter session duration. Such statistics negatively affect your ranking on Google. That’s the importance of the website’s technical elements.
Here, we enumerated components that affect your website’s technical structure:
- On-page SEO: Optimize meta tags like title tag, description, and headers. These are integral search index elements. Google uses title tags to crawl and index relevant results.
- Structured data: Rich snippets provide concise information to the ranking systems. For example, eCommerce pages use snippets for more detailed product information. They provide details like product names, categories, prices, and reviews.
- Multimedia optimization: File sizes of photos and videos are quite heavy. You might want to compress them into smaller file sizes. Use image compressor tools like TinyPNG or Optimizilla.
Be sure to check Google’s guidelines on technical SEO. They post regular updates regarding the technical aspects of site indexing.
Social signals
Social signals are engagement metrics from social media platforms. The more people engage with your posts, the higher your social signals are.
But why is a social signal considered a ranking factor? Isn’t social media a different platform from Google?
Yes, social signals don’t directly influence ranking systems. However, take a look at this sequence to discover how important social signals are:
- You publish a social media post with inbound links to your website.
- Your post gathers thousands of likes and hundreds of engagements.
- Since your post is viral, people become curious about the link you included.
- Traffic from that social media post increases drastically.
That’s the influence of social media on SEO. It’s a free word-of-mouth marketing channel. Follow these tips to encourage social engagement:
- Use social listening tools to understand your audience. These tools track trending topics on social media platforms. Sprout Social is one example of a social listening tool.
- Create visually appealing content. Don’t rely on heavy text. Infographics and videos are more shareable content forms.
- Work with influencers in your niche. Influencer marketing can significantly boost your campaigns. Just make sure to collaborate with reputable and industry-relevant influencers.
Social media does help you rank higher on search results. Even more, it can help generate backlinks to your site.
User engagement metrics
We mentioned bounce rate and session duration in the previous sections. These are examples of user engagement metrics. They make up an essential ranking factor for websites.
First of all, these metrics tell how much your audience likes your website. This includes your content, interface, and loading speed. Regularly monitor user engagement metrics, especially these ones:
- Bounce rate: How many visitors are leaving your website after just one page?
- Dwell time: How long do users spend viewing your website?
- Click-through rate: How many users click on your link in search results?
Google Search Console is a good place to get started. This free tool offers insights into user engagement metrics and core web vitals. Analyze these metrics and optimize low-performing pages.
Keeping Up with Google Algorithm Updates
Staying updated with Google algorithms is essential. In fact, it’s one of the top Google ranking factors. Typically, Google releases monthly updates to their algorithms.
However, there are major algorithm updates that need more attention. Here are some recent ones:
- October 2023 Spam Update: This update targeted spammy SEO tactics. Google punished websites with spammy internal links pointing to certain pages. They also enhanced the AI-based spam-prevention system SpamBrain.
- March 2024 Core Update: This core update introduced the helpful content system. It encouraged websites to prioritize search intent and unique content. This changed how websites do keyword optimization and other on-page SEO strategies.
There are resources that’ll help you stay updated. You can check reputable publications like Search Engine Journal. Moreover, SEO tools like Semrush and Moz provide insights on algorithm updates.
Strategies for Improving Google Search Results
Let’s explore practical strategies you can use to drive more organic traffic on Google.
Keyword research and optimization
Researching relevant keywords is a big part of your content playbook. However, there are times when your competitors target the same keywords. How can you separate yourself from the pack?
There are certain strategies that could give you an advantage. These tips can help you optimize keywords better:
- Content differentiation: Look for content forms that your competitors haven’t produced yet.
- Local SEO: Optimize keywords and content for local customers. This is particularly helpful for eCommerce websites.
- Voice search: Utilize keywords in conversational contexts. This helps you target search intent even better.
- Performance monitoring: Track the performance of your keywords. Serpstat is an excellent tool for monitoring keyword performance on search and paid ads.
Moreover, make use of marketing tools. These platforms gather useful information from user search history and patterns. Google Analytics and Marketo are powerful tools that offer such solutions.
Ultimately, we recommend listening to your audience. Pay attention to their comments and feedback. This gives you unique information that keyword tools can’t offer.
Content marketing and SEO
The ranking systems don’t just reward pages based on content length. They certainly don’t just look at how many keywords are incorporated.
Instead, it’s about the quality and relevance of your content.
Quality content improves organic traffic and domain authority. In turn, this gets you in the good graces of Google’s ranking systems.
Optimizing your content is a continuous process. Start with these proven strategies:
- Leverage different content formats. Some audiences prefer videos, while others want something they can read. Experiment with content types to gauge your audience’s interests.
- Repurpose and update your existing content library. You can turn long-form blogs into engaging reels and videos. Most importantly, update information and facts to guarantee your content’s accuracy.
- Cross-promote your content. For example, you can share social buttons on your website. This could encourage people to share your content.
- Regularly check Google’s content policies. You should know what you can and cannot publish on your website.
Moreover, be consistent in your content rollouts. Create a content schedule to keep up with your strategy. Use content scheduling tools like Asana or CoSchedule.
Link building and outreach
Link building is one of the top priorities when improving a website’s authority. Once other websites see your value, the backlinks will come pouring in.
Still, take note that link-building is a long-term strategy. You’re here to form relationships in the most natural way possible. You don’t just request or purchase links right from the get-go.
Here’s how you can establish relationships through link-building:
- Engagements: Don’t just directly reach out to a potential partner. Engage with their content by commenting and sharing their posts. Then, you can start offering avenues for future partnerships.
- Personalization: Refrain from generic messages or emails. Address how you can specifically help their audience. This way, they’ll know that you really did your research.
- Broken link building: Identify a broken link on another website. Then, offer to write content as a replacement. Too many broken links are a problem for many websites. They’ll be happy to take you for your offer.
Furthermore, always track your link-building progress. Avoid low-quality links from unrelated websites. Also, avoid too many outbound links to low-authority websites.
Technical SEO and site health
Your website’s technical health is as important as any SEO element. Google is big on providing a smooth user experience.
Knowing what to do during technical issues is key. Yet, we recommend avoiding such problems in the first place. Prevention is better than cure, as they say.
Follow these tips to ensure the good health of your website:
- Site audits: Regularly conduct site audits throughout the year. Typically, we recommend a quarterly or bi-monthly audit cycle. Also, you might want to monitor changes when there are algorithm updates.
- Link health: Check the status of your internal and outbound links. See if your internal link anchor text incorporates relevant keywords.
- SEO tools: Leverage digital tools and platforms to your needs. Make the most out of free tools or product demos. For example, Screaming Frog is an audit tool that crawls 500 URLs for free.
Many websites also work with digital marketing agencies to ensure website health. There are many moving parts in the technical aspect of a website. It might be an overwhelming task for a company’s internal team.
For instance, there are agencies like Fortis Media that offer year-round SEO auditing. This way, you don’t have to worry about any technical issues on your website. They’ll set up real-time alerts and a dedicated account manager for you.
Key Takeaways
Ranking on the first page of Google requires a long-term approach. It’s impossible to optimize every SEO aspect in just one go. That’s why you should monitor the ins and outs of your website.
Knowing the strengths and weaknesses of your website is a minimum requirement. Regularly conduct SEO audits and monitor your campaigns’ performances. Consulting with SEO agencies could be a big help, too.
Most importantly, stay on top of evolving algorithms. Be ready to adapt to necessary changes in your SEO strategy. Prompt optimization is just as important as planning your SEO playbook.
FAQ
What determines your Google ranking?
Google ranks websites according to their technical, on-page, and off-page SEO aspects. These include essential areas in content strategies, keyword optimization, backlink profile, website loading speed, and mobile responsiveness. Moreover, Google monitors user experience statistics like bounce rate, dwell time, and click-through rate.
How does Google determine page relevance?
Google determines a web page’s relevance through its content, domain authority, technical health, and user behavior signals. Still, content is a priority for Google’s algorithms and search engines. Content should target relevant keywords and exhibit a semantic understanding of user queries.
How important are internal links for SEO?
Internal links are essential for websites because they serve as navigational points for both users and search engine crawlers. Faulty internal linking could lead to inaccurate indexing, which could negatively affect search engine rankings and traffic. Optimize internal linking through a clear website architecture and hierarchy.
How does Google handle duplicate content?
Google handles duplicate content through factors like canonicalization, content clustering, user experience, and search intent. Google doesn’t automatically penalize duplicate content, although it does prefer unique versions of a web page. To avoid such issues, use canonical tags or no-index tags.
What are Google’s guidelines for backlink quality?
Relevance and quality are at the forefront of Google’s guidelines for backlink acquisition. Google rewards websites that naturally acquires high-quality backlinks from other authoritative sites. It is encouraged to build backlinks through creating helpful content that addresses user queries and intent.
Read our other articles
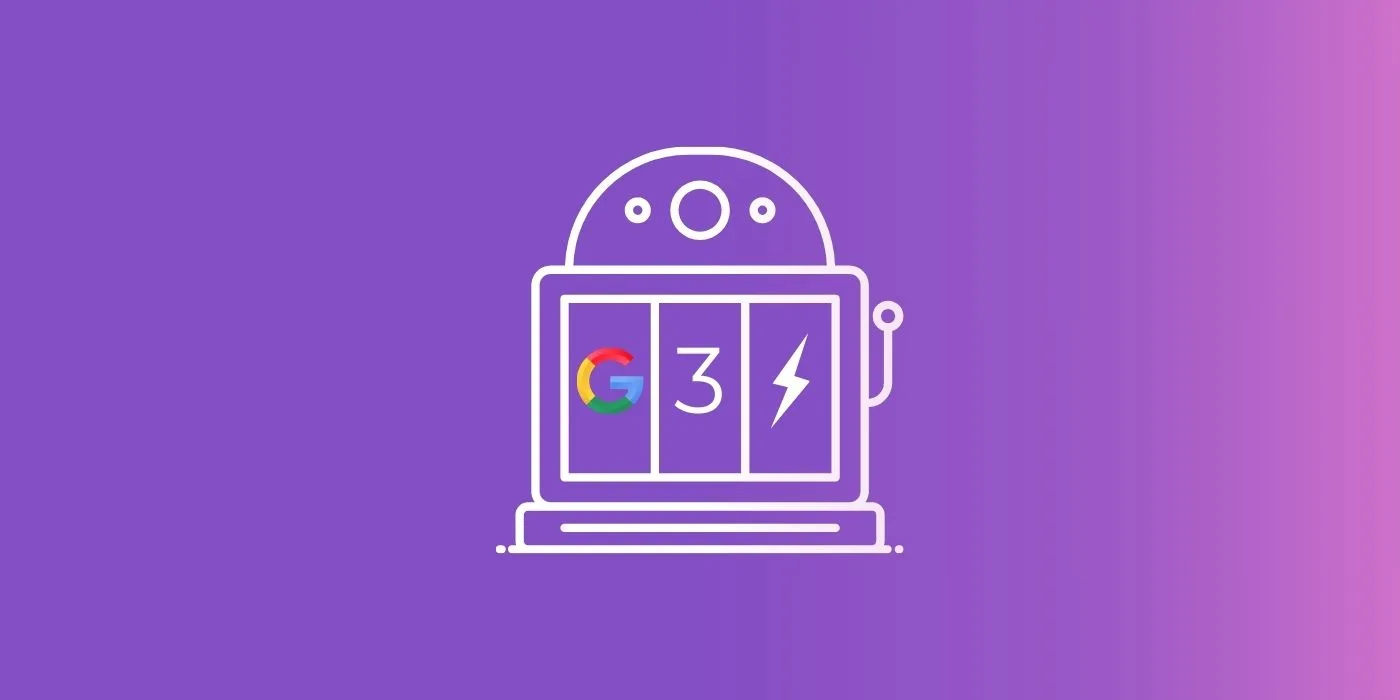
iGaming SEO: The Ultimate Guide to Ranking iGaming Sites in 2025
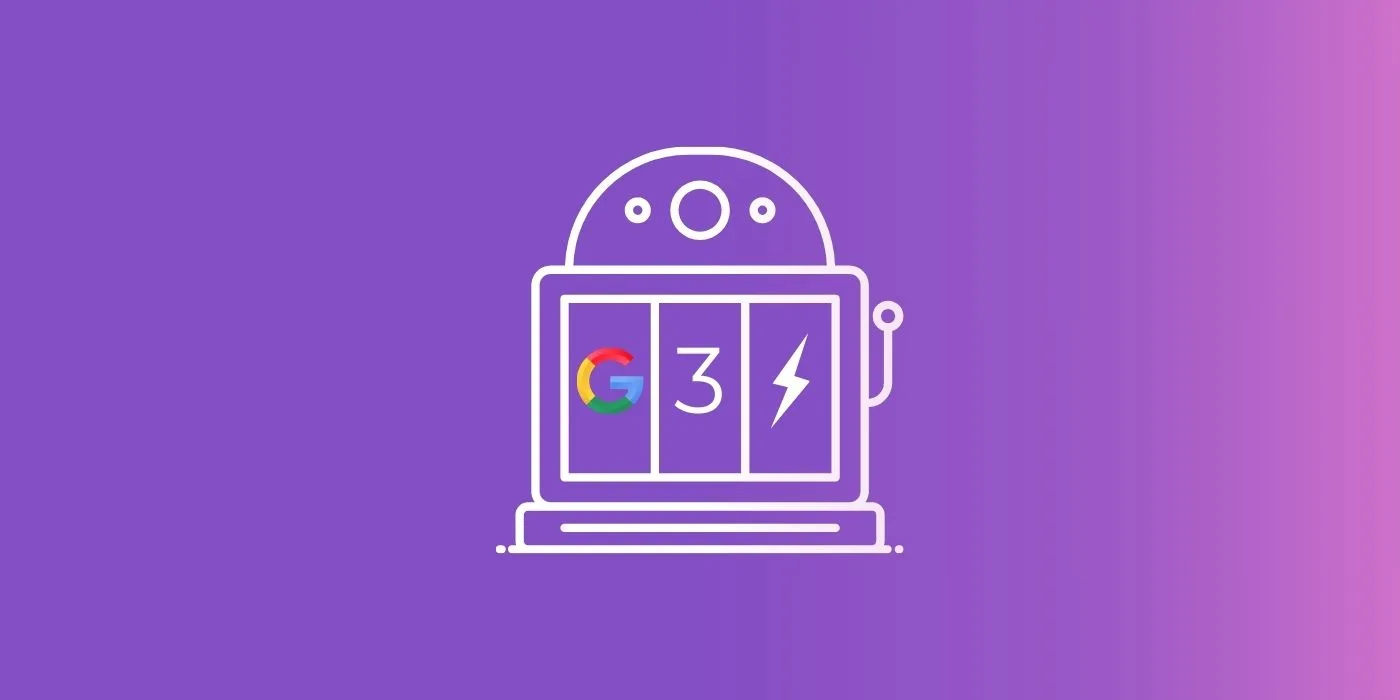
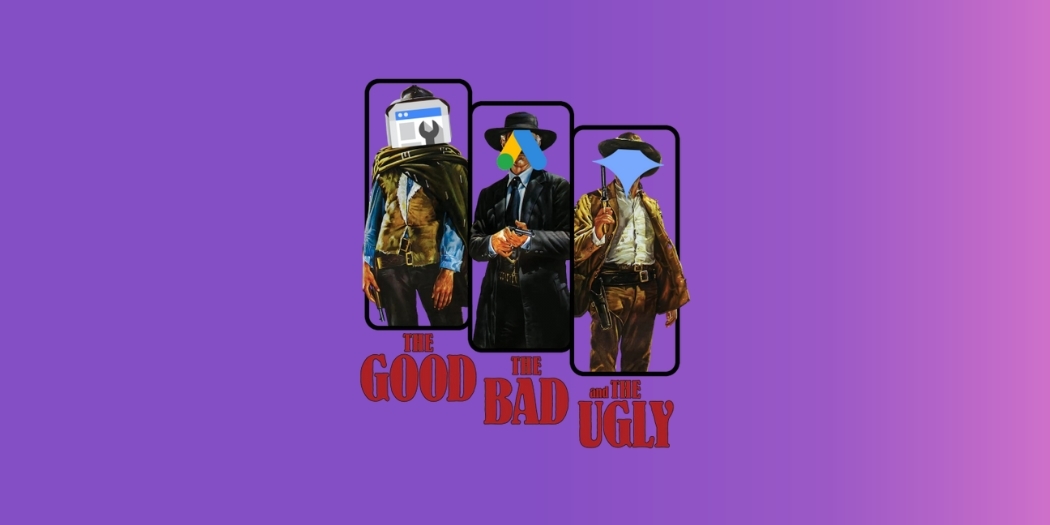
How SEO and PPC Work Together: A Practical Guide
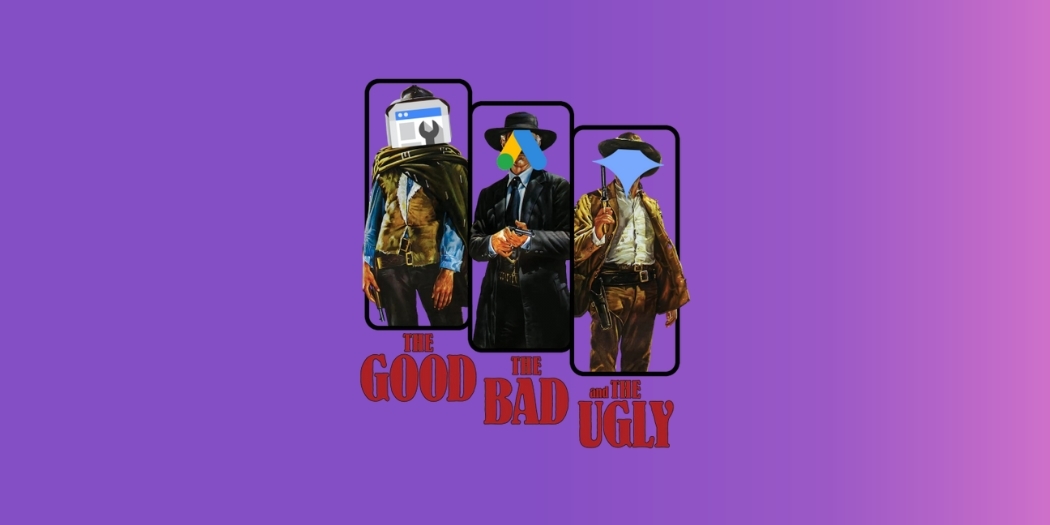
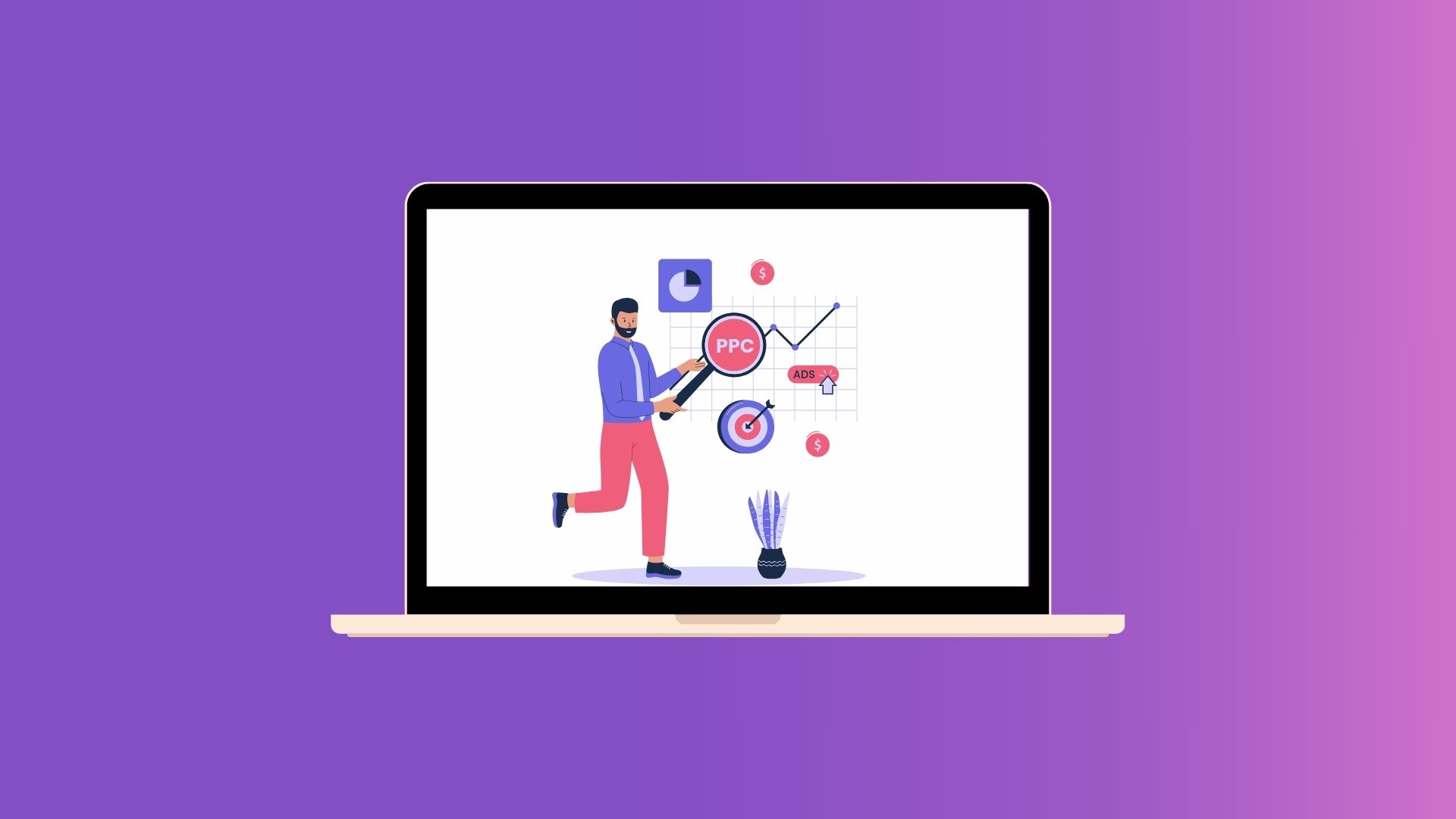
What is a PPC Agency? A Comprehensive 2025 Guide
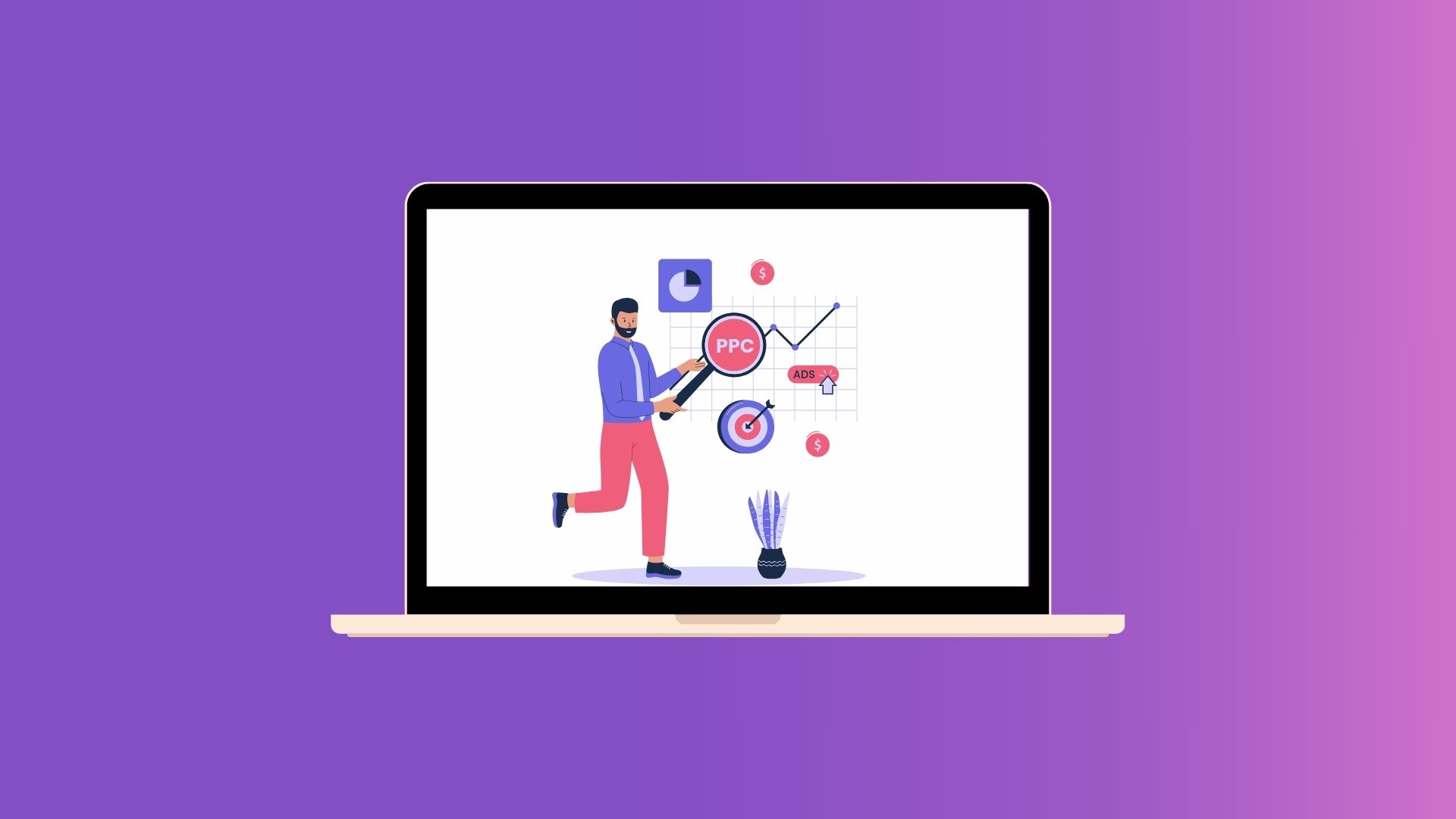